16 AI terms business leaders should know & understand
A knowledge of AI terminology is essential for modern business leaders
Add bookmarkListen to this content
Audio conversion provided by OpenAI
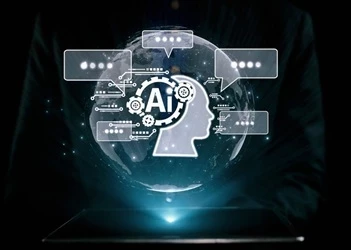
Artificial intelligence (AI) is evolving rapidly as technological advancements introduce vast new possibilities. Organizations of all sizes are increasingly investing in, using and developing AI to streamline operations, enhance efficiency and cut costs.
Growing adoption of AI is coupled with a stark diversification of AI technology as new capabilities become available. Take, for example, the emergence of generative AI in 2022 and, more recently, agentic AI – both of which have signaled dramatic shifts in the AI landscape.
The changing nature of AI along with its expanding role in modern businesses highlights the importance for leaders to both know and comprehend the various terms and phrases that define the technology.
AI is here and, as a business leader, you need to keep up, says Bob Gourley, chief technology officer (CTO) at OODA and author of The Cyber Threat. “A knowledge of some basic AI terminology could be the difference between being at the head of the pack or behind the curve. I’ve worked with top tech organizations, from startups to the government and have seen how understanding AI can make or break an organization.”
Here are 16 AI terms that enterprise leaders should understand as they traverse the ever-advancing AI landscape.
Don't miss any news, updates or insider tips from PEX Network by getting them delivered to your inbox. Sign up to our newsletter and join our community of experts.
1. Generative AI
Generative AI refers to deep-learning models that generate high-quality content based on the data they were trained on. Across industries and job functions, generative AI is transforming organizations by turning vast amounts of data into actionable knowledge, helping employees work more efficiently. For example, generative AI is ushering in a new age of operational excellence (OPEX), finding exciting applications across various settings including customer service, manufacturing, product development, supply chain, finance, human resources and IT support.
“Generative AI has the potential to transform OPEX by automating complex tasks, optimizing processes and enhancing decision-making capabilities,” says Anurag Lal, former director of the US National Broadband Task Force under the Obama administration and CEO of NetSfere. Generative AI can analyze vast amounts of data quickly, identifying inefficiencies while suggesting improvements, leading to significant cost savings and an increase in productivity.
2. Large language models (LLMs)
LLMs are text-based models that have been trained on all major literary works and large volumes of conversational data. They are the engines that power AI chatbots and virtual assistants. “Business leaders should understand how LLMs will transform internal processes and customer communications this year,” says Greg Squibbs, founder of Start Your AI Agency.
Watch thought leader Vishal Bhalla discuss the pros and cons of AI
3. Agentic AI
Agentic AI uses sophisticated reasoning and iterative planning to autonomously solve complex, multi-step problems. Agentic AI systems ingest vast amounts of data from multiple data sources and third-party applications to independently analyze, develop strategies and execute tasks. It uses a four-step process for problem-solving: perceive, reason, act and learn.
Forrester Research found that 90 percent of businesses expect agentic AI to give them a major competitive edge within the next five years. Meanwhile, Gartner predicts that, by 2028, 33 percent of enterprise software applications will include agentic AI, up from less than 1 percent in 2024. This will enable 15 percent of day-to-day work decisions to be made autonomously.
Agentic AI is driving investment to address complex business workflows. Meanwhile, it is also giving rise to the concept of agentic process management. This is an approach that utilizes the power of agentic AI to enhance process management.
“What makes agentic AI so powerful is its ability to solve complex problems while continuously learning and adapting,” says Erik Wikander, CEO and co-founder of Wilgot.ai. “Unlike traditional software, it doesn’t need manual updates or corrections, instead it works autonomously. The real potential of AI agents is fully replacing manual labor, not only making work processes more efficient.”
Register for All Access: Agentic AI 2025
4. AI agents
AI agents, on the other hand, are typically built to do specific tasks. Great at automating simple, repetitive processes, AI agents lack the autonomy or decision-making capabilities of agentic AI. They can be deployed in various applications to solve complex tasks in diverse enterprise contexts from software design and IT automation to code-generation tools and conversational assistants. OpenAI CEO Sam Altman predicts that AI agents will soon replace software engineers.
5. Foundation models
Foundation models are the large, pre-trained AI models that can learn lots of different things. They form the backbone of many AI applications, from language to image manipulation. Examples include ChatGPT or Google’s Gemini.
“A unique feature of foundation models is their adaptability,” according to AWS. “These models can perform a wide range of disparate tasks with a high degree of accuracy based on input prompts.” Some tasks include natural language processing (NLP), question answering and image classification.
The size and general-purpose nature of foundation models make them different from traditional machine learning models. “Foundation models, even though are pre-trained, can continue to learn from data inputs or prompts during inference,” AWS adds. “This means that you can develop comprehensive outputs through carefully curated prompts.”
6. Edge AI
Like edge computing, edge AI refers to the deployment of AI algorithms and models directly on local edge devices such as sensors or Internet of Things (IoT) devices, enabling real-time data processing and analysis without constant reliance on cloud infrastructure. Edge AI is growing in popularity as industries discover new ways to harness its power to optimize workflows, automate business processes and unlock new opportunities for innovation. It enables localized decision-making that eliminates the need to constantly transmit data to a central location and wait for the facilitation of automating business operations.
“This is becoming common where fast responses are needed and when it must always be on even without an internet connection as with self-driving cars,” says Squibbs. “This is also now widely used in industries like healthcare and retail.”
Watch Dan Riley, co-founder and CEO of RADICL and founding member of Society for People Analytics (SPA), reflect on integrating AI into decision-making processes
7. AI hallucinations
AI hallucinations are when a large language model (LLM) – typically a generative AI chatbot – creates outputs that are nonsensical or altogether inaccurate. These can be the result of a variety of factors such as training data inaccuracy, high model complexity and even malicious interference. AI hallucinations include incorrect predictions, false positives and false negatives. They can have significant consequences for businesses, particularly if outputs are not verified for accuracy and correctness. AI hallucinations may result in legal, ethical and economic issues, dismantling trust in the technology and the establishments that implement it.
“There are checks and balances available now with technology getting more mature to handle this, but still, there is no foolproof guarantee that the answers you’re getting are not hallucinated or that it’s really the answer you wanted,” warns Priyank Mangal, senior director at Edgeverve.
Register for All Access: AI in PEX 2025
8. AI bias
AI bias refers to prejudiced AI-generated results influenced by human biases that skew original training data or algorithms. AI bias can occur in various stages of the AI pipeline, with a common source being data collection. Others include data labeling, model training and deployment. Types of bias can range from selection and confirmation to measurement and stereotyping.
Similar to hallucinations, AI bias can lead to distorted output and potentially harmful outcomes. Biases can lead to severe repercussions, especially when they contribute to social injustice or discrimination. This is because biased data can strengthen and worsen existing prejudices, resulting in systemic inequalities. Hence, it is crucial to stay alert in detecting and rectifying biases in data and models and aim for fairness and impartiality in all data-driven decision-making processes.
9. AI governance
AI governance relates to processes, standards and guardrails that help ensure AI systems and tools are safe and ethical. Driven by research, development and application, AI governance frameworks are essential for complying with increasingly strict regulations, laws and standards surrounding AI use. It is also integral to trust and responsibility in developing and applying AI technologies.
“Effective AI governance includes oversight mechanisms that address risks such as bias, privacy infringement and misuse while fostering innovation and building trust,” states IBM. An ethical AI-centered approach to AI governance requires the involvement of a wide range of stakeholders including AI developers, users, policymakers and ethicists, ensuring that AI-related systems are developed and used to align with society’s values.
Watch Tyrone Smith Jr, program faculty member/adjunct associate professor at University of Southern California (USC) and founding member of SPA, discuss building trust in AI
10. AI security
Like AI governance, AI security is concerned with the safe and controlled use of AI. Whereas governance focuses more on meeting standards and ethical guidelines, security focuses on risks specific to AI technology, including cyber attacks and data breaches. AI renders cyber attacks increasingly difficult to defend against. Meanwhile, threat actors are becoming more intelligent, and they’re using AI to do it.
There are considerable security and leakage risks associated with AI use and implementation, says Lee Bogner, global AI and generative AI chief enterprise architect at Mars Inc and member of the PEX Network Advisory Board. “Vulnerabilities to data leakage and cyber-attacks may come from direct prompt injections, indirect prompt injections, adversarial attacks, exploits and so-called jailbreaks,” he adds. “As a result, these models are inherently leaky and require industriousness to align with organizational values and user goals.”
11. Retrieval augmented generation (RAG)
RAG is a technique for enhancing the accuracy and reliability of generative AI models with information from specific and relevant data sources. It allows a business to combine the most up-to-date and relevant information it has with an AI model in order to provide more accurate and timely responses. “Think of it as a way for AI to look up additional information specific to the user to improve the quality of its response,” says Dom Couldwell, head of field engineering EMEA at DataStax.
RAG gives models sources they can cite, help models clear up ambiguity in a user query and reduces the possibility that a model will give a very plausible but incorrect answer (hallucinations).
12. Explainable AI (XAI)
This is AI that can explain how it comes to decisions. Its main purpose is to provide humans with the ability of intellectual oversight over AI algorithms, making them more understandable and transparent. AI researchers have identified XAI as a necessary feature of trustworthy AI, and explainability has experienced a recent surge in attention.
“If you’re using AI to make significant decisions for your business, you’ll want to know why it decided on something,” says Gourley. “Explainable AI gives you its calculations, so you will not be surprised by its decisions.”
13. Multimodal AI
The concept of multimodal AI refers to systems that can process and integrate different types of data – text, images, audio – all at once. “It’s a turning point for industries like healthcare, where multimodal AI can combine patient records, imaging scans and real-time monitoring to provide faster diagnoses,” says Ayush Trivedi, CEO of Cyber Chief.
It can be thought of as giving AI the ability to process and understand different sensory modes, meaning users are not limited to one input and one output type and can prompt a model with virtually any input to generate virtually any content type.
14. Synthetic Data
Sometimes real data is unavailable or too personal to use to train AI. Synthetic data is fake data that acts just like real data. It looks and feels the same as original data, but the big advantage is that the synthetic data does not contain personal information.“It is a great way of training AI without needing to worry about privacy concerns,” says Gourley.
Watch Lee Bogner, global AI and generative AI chief enterprise architect at Mars Inc., discuss the importance of data in AI
15. Federated Learning
This is where AI learns from data without actually sharing it. It doesn't require an exchange of data from client devices to global servers. Instead, raw data on edge devices is used to train the model locally, increasing data privacy. For example, if companies have private customer information, they can use that information to train AI without relinquishing it. Federated learning is becoming the standard for meeting a raft of new regulations for handling and storing private data.
16. Neuro-symbolic AI
This is AI that has the capability to reason both logically and creatively. It’s a mixture of computers’ intelligent learning and human-like reasoning. This AI makes it possible for complicated business issues to be resolved in more intelligent ways.
All Access: Change Management or Business Transformation 2025
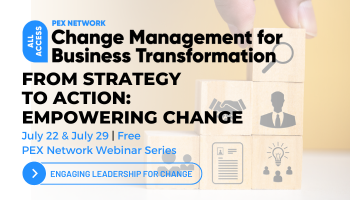
All Access: Change Management or Business Transformation 2025 from PEX Network will help professionals get an understanding of the latest developments in change management, including how to overcome resistance to change, how to create a culture of change, and how to measure the success of change initiatives.
Register Now