Addressing AI bias & ensuring fairness in systems
Tackling AI bias is not just an ethical concern – it is a business necessity
Add bookmarkListen to this content
Audio conversion provided by OpenAI
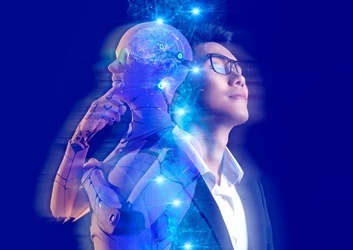
Artificial intelligence (AI) is transforming industries with AI-driven decisions impacting financial access, healthcare diagnoses, hiring and more. If left unchecked, biased models can reinforce discrimination, damage reputations and lead to regulatory penalties.
Ensuring fairness in AI is not just an ethical concern – it is a business necessity
Don't miss any news, updates or insider tips from PEX Network by getting them delivered to your inbox. Sign up to our newsletter and join our community of experts.
Understanding AI bias
Bias in AI typically has three primary sources. One of the most common is data bias when historical or real-time data inaccurately represents the target population or is skewed due to systemic issues (e.g. underrepresentation of specific demographics).
Algorithmic bias can appear even with representative data, as certain modeling choices or inherited assumptions can lead to unfair outcomes. For instance, over 60 percent of AI-driven hiring tools exhibit bias, perpetuating discrimination in recruitment.
Last but not least is human bias – when people embed their prejudices into the system during designing, labeling or selecting data.
Fairness in AI is about excluding systematically disadvantaging or favoring certain groups. Such industries as finance, healthcare, human resources and marketing are more likely to face such risks. These sectors directly affect people’s livelihoods and well-being – and regulators are increasingly focusing on bias mitigation. Some frameworks focus on equal opportunity (ensuring consistent performance across demographic groups), while others emphasize parity in outcomes (e.g. similar acceptance rates).
The importance of AI transparency
According to research, 78 percent of organizations adopted AI by 2024, but employees nevertheless display significantly less enthusiasm about using AI in their daily activities. The common challenge is called the “black box” problem, where it is unclear how AI models make decisions.
Transparency is achieved through explainable models, documentation, clear communication and constant audits. By prioritizing visibility at each stage of development and deployment, organizations can mitigate the black box effect often associated with AI systems and allow non-technical stakeholders to evaluate system fairness.
At IBA Group, we use a multi-step approach to detect and reduce bias. This starts with data audits to detect underrepresented or skewed samples. To track bias metrics, we use algorithmic auditing tools such as IBM’s AI Fairness 360 or Microsoft’s Fairlearn. Model interpretability techniques such as SHAP and LIME help us understand how model predictions relate to sensitive attributes.
We also refine model architectures with iterative model tuning to reduce biased outcomes. We engage subject matter experts and, in some cases, end users to confirm the fairness and relevance of final models.
Regulations for ethical AI
The EU AI Act takes a strict risk-based approach, banning especially harmful AI practices and imposing heavy requirements on “high-risk” AI systems (like those used in hiring, lending or medical diagnostics). For instance, under the EU AI Act, failing to comply with prohibited AI practices can mean fines of up to EUR 35,000,000 or 7 percent of worldwide annual turnover, whichever is higher.
In the US, a similar framework comes from the National Institute of Standards and Technology (NIST). It provides a roadmap for organizations to identify and manage AI risks, focusing on principles like safety, accountability and fairness (it even includes “managed bias” as a core theme). Beyond these, the OECD AI Principles (adopted in 2019 by 47 governments) have been highly influential, setting broad values for AI like fairness, transparency and human-centricity, which many national strategies and other frameworks echo.
Industry groups and standards organizations are also active: the IEEE has been developing ethical AI standards, for example – guidelines on algorithmic bias and AI system design transparency. Additionally, other regions are moving forward. China has released regulations on recommendation algorithms and deepfakes and Canada introduced an Algorithmic Accountability Act for public sector AI.
Big tech firms like Microsoft have already adopted the NIST AI RMF and soon it will become a common benchmark. Meanwhile, McKinsey’s 2025 report notes that only 1 percent of companies believe they have achieved AI maturity, implying most lack robust governance frameworks
The future of fair and ethical AI
Over the next five years, the primary driving force behind AI and machine learning adoption will continue to be clear economic benefits. At the same time, fairness and bias mitigation will increasingly be recognized as “must-have” secondary objectives rather than purely altruistic considerations.
Companies must implement rigorous pre- and post-training data filtering, ensuring biased data is removed or adjusted before it influences AI outputs. Continuous model monitoring helps detect unintended biases, while human-in-the-loop approaches introduce expert oversight to correct problematic outputs. Establishing clear usage policies for generative AI applications further minimizes risks.
Overall, AI adoption will be shaped by a “business-first but fairness-considered” mentality – with the expectation that straightforward bias-mitigation solutions will be part of the standard AI toolkit, ensuring organizations can easily adapt AI’s financial upside without neglecting ethical concerns.
As the technology matures, community and regulatory scrutiny will further drive improvements in bias detection and mitigation.
All Access: Change Management or Business Transformation 2025
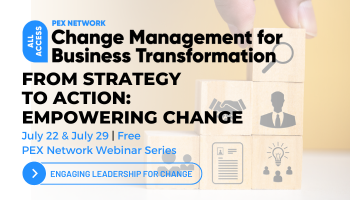
All Access: Change Management or Business Transformation 2025 from PEX Network will help professionals get an understanding of the latest developments in change management, including how to overcome resistance to change, how to create a culture of change, and how to measure the success of change initiatives.
Register Now