Why AI isn’t a green technology for data operations
DataOps is increasingly used by businesses to gain more value from their information to empower optimization
Add bookmark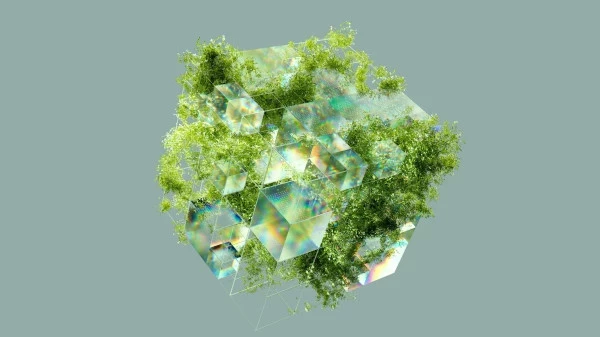
Artificial intelligence (AI) and sustainability are two of the hottest topics in business transformation right now. The rapid advancement of AI (cue Generative AI) is a game-changer for so many aspects of business process and operation optimization as well as transformation. Meanwhile, modern organizations are under increasing pressure to reach sustainability and carbon footprint reduction goals to not only meet customer expectations and regulatory requirements but to also drive revenue and profit opportunities.
Data operations (DataOps) refers to a set of practices, processes and technologies that combine an integrated and process-oriented perspective on data with automation and methods from agile software engineering to improve quality, speed and collaboration, promoting a culture of continuous improvement in the area of data analytics.
With the potential to make data agile, accurate and efficient from end to end, DataOps is increasingly being applied by businesses to gain more value from their information to empower optimization. However, data infrastructure and DataOps processes can be very energy intensive. AI technology is seen as one potential source for helping to reduce DataOps emissions by using fewer resources, but according to Roman Khavronenko, co-founder of VictoriaMetrics, organizations should not deceive themselves that AI is currently a green technology for DataOps emissions.
PEX Network speaks to Khavronenko to find out more.
PEX Network: What are AI’s limitations as a DataOps green solution?
Roman Khavronenko: Many of the limitations around AI are due to the infancy of the technology. While AI adoption has been rapid, the technology was barely ready for market before models started being developed for commercial use. In the future, when efficiency improves and the costs come down, AI could be used for tasks that require constant monitoring, like power management or resource allocation.
Currently, there are automated solutions that fulfil these functions, but a more “intelligent” solution could find efficiencies dynamically that current options are not sophisticated enough to manage. To use a very broad example, the internet uses somewhere between 84 and 143 gigawatts of electricity. If AI tools could achieve just a 2% efficiency gain then it would be comparable to a small country using 100 percent renewable energy. The limitation of this is that the cost of training current AI tools would not be more efficient than the energy savings such a tool could generate.
PEX Network: Why is AI often heralded as a data sustainability panacea?
RK: It comes down to responsibility and complexity. The goal of achieving sustainability is incredibly complex and requires all stakeholders to take ownership of the problem. Unfortunately, AI is perceived by some in business as a way to transfer responsibility. The scale of the problem is almost at the limits of human comprehension and represents a massive challenge for every business that is meaningfully attempting to tackle emissions.
Rather than directly tackling sustainability and thereby running the risk of failing or coming up short, an AI tool can handle the task of monitoring and enhancing efficiency. If the tool fails, then blame is levied at the platform rather than the corporation or individuals that oversee the tool. In theory, the eventual capabilities of AI are limitless, and many companies are pinning their sustainability hopes on a technology that has not yet manifested. This exonerates those in the present from their responsibilities to curb emissions.
PEX Network: How can businesses make existing options more efficient to drive down DataOps emissions?
RK: It’s important not to understate the importance of legislation in shaping and regulating the market. Latency issues and certain data storage requirements mean that governments have latitude in how legislation creates a market that rewards efficiency. GDPR and similar regulations mean that a harsher regulatory environment won’t drive as many data centers to move to other locales.
The first step will be to set goals for companies to achieve. Once the goal is established, DataOps companies will begin evaluating their hardware and software stacks to identify potential efficiencies that were previously overlooked. Some data centres already carbon offset by using coolant water to run heat pumps, reclaiming some of the energy for domestic or industrial use.
For a purely business focused answer, optimizing the efficiency of data processing operations is key. This can involve refining algorithms for better performance with less computational overhead, using more energy-efficient hardware and adopting cloud solutions that allow for more efficient resource utilization. In theory, a hyperscale data center can access efficiencies of scale that are not an option for most, but the decision to pursue these efficiencies will be a purely economic one, and that is why governments need to set the tone here.
Interested in presenting your own Case Study?
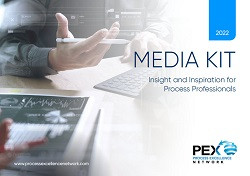
Share best practice, ideas, and solutions to our global community of over 165,000 senior process professionals.
View our latest Media Kit to learn more about our audience and review our content calendar. Download Now
Learn More