The guide to AI in operational excellence
How AI is revolutionizing operational excellence and driving efficiency
Add bookmark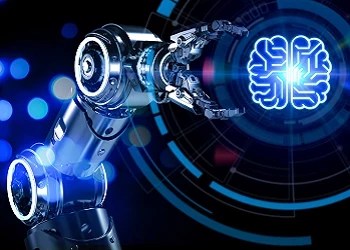
The goal of operational excellence is to make an organization's operations more efficient, while minimizing waste and costs and continually improving. The ability of artificial intelligence (AI) to streamline workflows, analyze complex data and provide insights to support decision-making makes it a valuable tool for operational excellence initiatives.
In this guide, we explore the capabilities of AI and subfields like machine learning (ML) and automation, how businesses are using these to drive efficiency and factors you should consider during implementation like change management, ethical and regulatory implications.
Don't miss any news, updates or insider tips from PEX Network by getting them delivered to your inbox. Sign up to our newsletter and join our community of experts.
Contents
- AI, machine learning and other subsets
- How does AI enhance operational excellence?
- What practical functions does AI have?
- AI adoption and change management
- Regulatory and ethical considerations
- The future of AI in operational excellence
AI, machine learning and other subsets
First, it’s worth briefly clarifying the difference between AI, ML, deep learning and neural networks. These are often used interchangeably, but the latter three are in fact subsets of AI.
AI is the simulation of human intelligence processes by machine and computer systems. It encompasses these subfields:
- Neural networks: A type of AI algorithm inspired by the structure and function of the human brain consisting of interconnected neurons. Neural networks can be trained to recognize patterns and relationships in data, enabling them to perform tasks such as classification and pattern recognition.
- ML: A subset of AI that focuses on developing algorithms and models that learn from data to make predictions or decisions, instead of relying on predefined rules.
- Deep learning: A subset of ML that uses neural networks with many layers, enabling the processing of large amounts of data and recognizing patterns in pictures, text and sounds to produce complex models.
- Natural language processing (NLP): NLP is another branch of AI that enables machines to understand, interpret and generate natural-sounding language.
- Robotics and automation: The design and programming of robots and automation to perform tasks traditionally done by humans, incorporating AI.
All of the above are being used increasingly to improve business operations, as we’ll see below.
How does AI enhance operational excellence?
AI is increasingly used as a cornerstone for boosting efficiency across business operations, complementing the key operational excellence concepts of Lean, Six Sigma, business process management (BPM), total quality management (TQM) and continuous improvement. Here is how it can enable each of these:
- Lean: By analyzing vast datasets, AI identifies inefficiencies and waste, helping organizations make data-driven decisions that align with Lean principles.
- Six Sigma: AI algorithms can detect patterns and anomalies in data that lead to variations in manufacturing or service processes. By identifying these, defects can be removed and higher levels of quality control are achieved in line with the Six Sigma methodology.
- BPM: AI enhances BPM by automating tasks, enabling predictive analytics, optimizing processes and enabling adaptive process management. By integrating AI technologies into BPM initiatives, businesses can achieve higher levels of efficiency and agility.
- TQM: TQM is focused on continuously improving the quality of products, services and processes within an organization, while maintaining customer satisfaction. AI supports this by analyzing customer feedback and performance data, providing insights that enable organizations to continuously refine their processes and offerings.
- Continuous improvement: AI also facilitates continuous improvement by providing real-time monitoring and constant analysis of operational data.
What practical functions does AI have?
According to PEX Network’s 2024 Global State of Process Excellence survey, 35 percent of respondents plan to use AI this year. The top areas of deployment are in operations (27 percent), customer service (24 percent) and data processing (22 percent).
AI’s role in driving the operational excellence of an organization can take several forms, all of which are underpinned by data analytics.
Intelligent automation
While robotic process automation (RPA) uses programmable bots to emulate the human execution of business processes, intelligent automation (IA) goes further by using AI and machine learning in combination with process mining, advanced analytics and other technologies to turn business processes into autonomous systems that can make decisions independently.
Logistics company Maersk implemented this within its cash collections process. Algorithms were run on data to learn from customer behaviors, using this to predict whether they will pay on time and if they need reminders. As well as assessing the level of risk in selecting customers, it was then able to predict cash flow based on these insights.
Predictive maintenance
Predictive maintenance is a clear example of the intersection of AI and Lean. Machine learning algorithms can analyze historical data to predict the maintenance needs and potential failures of equipment. By identifying issues before they become major problems, maintenance can be scheduled ahead of time to prevent unplanned downtime and cut costs.
When Toshiba used this approach it found this improved the performance of its equipment and extended its lifespan. As well as preventing expensive failures, it meant that equipment operates at maximum efficiency, reducing energy consumption and operating costs.
Supply chain optimization
AI can optimize supply chain operations, including demand forecasting, inventory management and logistics. Fashion retailer Zara embeds microchips into garments’ security tags and adds RFID tags onto packaging. Combined with an AI-powered data analytics platform, the company can manage its inventory and predict demand to ensure that popular items are always available.
Quality control
Thanks to their ability to understand visual data, deep learning algorithms have emerged as a highly efficient tool for detecting anomalies during quality control inspections. This can save hours of human labor, while identifying issues early means that organizations can take actions promptly to maintain high-quality standards and reduce waste.
At an Audi plant in Germany, AI is used for the quality control of spot welds, the parts of a vehicle where metal sheets are joined together during manufacture. This previously had to be done manually by employees who would draw samples at random for inspection – a far less efficient process.
Intelligent document processing (IDP)
IDP is a key component of operational excellence as it involves automating document-heavy processes by extracting relevant data and feeding it into systems or workflows. Conversational AI models can now make IDP far more intuitive to users thanks to NLP and ML. The AI can extract data, automate document workflows and gain insights by simply asking questions or issuing voice commands in natural-sounding language.
Digital transformation
Organizations that digitally transform can gain a competitive advantage and increase operational efficiency. AI, and more recently generative AI, play a significant role in supporting digital transformation initiatives by enabling data-driven decision-making, automating processes and enabling predictive analytics.
In the case of generative AI, it can accelerate software development by translating natural language into code, turning unstructured information into more structured formats and templates, and even helping teams generate test data.
READ: Eight more ways leading companies are using AI to drive operational excellence
AI adoption and change management
A carefully considered change management strategy is vital for any new technology to be successfully adopted. This is even more important in the case of AI, as due to its transformative potential there may be concerns from employees who fear job displacement or feel apprehensive about adopting new technology. Here are a few tips to manage the transition:
1. Communicate the benefits of AI
Provide clear communication of the benefits the new technology will bring, emphasizing its value to the business with examples of how it will improve efficiency, reduce errors and enhance job satisfaction.
2. Provide training and support
Make sure employees can access the training they need to develop the skills necessary to work alongside AI systems. This could involve upskilling or reskilling employees to take on more strategic and value-added tasks that complement AI capabilities.
We have compiled the seven best free AI training courses for business leaders here.
3. Involve employees in the AI adoption process
Involving the workforce in the AI adoption process fosters a sense of ownership and collaboration. Meet with teams regularly to ask for their input and address their concerns.
4. Redesign processes where necessary
You may need to redesign certain processes along with people’s roles and responsibilities to make the most of AI integration. For example, some tasks previously performed by people may become automated, while new tasks may emerge that require human supervision.
This article on embedding intelligent automation into organizational culture by the director for change management global services North America at Johnson & Johnson, offers a good explanation of the importance of a clear change management strategy.
Regulatory and ethical considerations
AI has very clear benefits in terms of operational excellence, but it also comes with regulatory and ethical considerations that businesses need to address. One important concern is data privacy, as AI systems rely on vast amounts of data, raising questions about the collection, storage and usage of sensitive information.
- Data privacy must be prioritized by implementing robust security measures, obtaining informed consent from individuals and adhering to data protection regulations such as the European Union’s GDPR or the US’ CCPA.
- Bias is another consideration in AI, as algorithms may perpetuate biases present in training data. This can lead to discriminatory outcomes in decision-making processes, an important aspect to consider in customer-facing sectors such as banking, for example. To prevent this, organizations must carefully design AI systems, regularly audit algorithms for fairness and diversify training data to ensure it is representative and inclusive.
- Transparency is essential for building trust in AI systems, as stakeholders need visibility into how decisions are made and what data is used. Organizations should strive for transparency by providing clear explanations of AI processes, disclosing potential limitations or biases and fostering open communication with users and stakeholders.
Last but not least, consider how adopting AI will impact your organization’s environmental, social and governance policies. While its capacity to increase productivity by using fewer resources is beneficial, it might lead to undesirable outcomes elsewhere. One example explained in this article is that AI should not be relied on as a green technology to reduce emissions from data operations.
The future of AI in operational excellence
The most immediate impact of AI is reducing costs, as it enables businesses to do more with less resources. Experts believe that AI-driven automation will increasingly be used across industries to automate routine tasks and free up human resources to focus on more strategic activities. According to McKinsey, generative AI could automate 25 percent of workplace tasks in the near future, and when combined with other enabling technologies such as workflow automation, it could potentially automate tasks that currently absorb 60-70 percent of employees’ time.
Generative AI will also become deeply embedded into many more aspects of business operations. As Ulrich Irnich, chief information officer of Vodafone Deutschland, explains, “Generative AI will be everywhere. It will be fully integrated into all interactions, process excellence, new AI process modeling and, eventually, will evolve into vertical specific languages.”
As AI continues to evolve and mature, businesses can expect to see increased integration of AI into their day-to-day operations, opening up new opportunities for efficiency and growth.
The Gateway to Enhanced Productivity, Cost Savings, and Organizational Growth
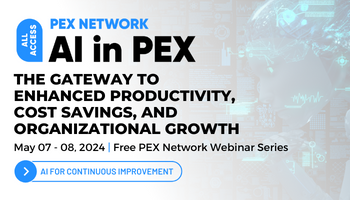
PEX Network's All Access AI in PEX series will show you how to use this bleeding-edge technology to improve your business performance and process excellence. You will learn from leading experts in the sector and discover the latest solutions and best practices for operational excellence and digital transformation. Register now and get access to this free PEX Network webinar series.
Register Now